About language, learning and tomatoes
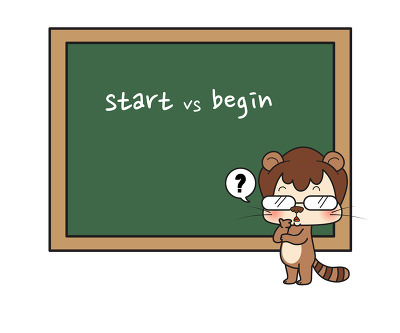
In our last blog we talked you through the Rescorla-Wagner rule and worked through a classic example from the literature. This week we would like to repeat this procedure on an actual linguistic example. Let's say that we want to use the same algorithm to learn when to use the verb start and when to use the verb begin from exposure to examples only. There are two forms to choose from: START and BEGIN. Native speakers of English won't find it hard to select the verb that fits the grammatical and semantic context best, but what guides them? Let's look at a few examples:
- The movie ______ at 7:30pm.
- The car wouldn't ______ .
- My grandfather _____ his company in 1927.
- The meeting ____ at 9am.
- We should ___ early, before traffic gets busy.
- The day _____ well.
Table 6 presents information just like the one that was given in Table 1, but this time for verbs. Let's agree to label the context that requires one of the two verbs we're interested in <commence>.
Table 6
Cues | Outcomes | Frequency |
commence; journey | START | 2 |
commence; machine | START | 11 |
commence; movie | START | 19 |
commence; movie | BEGIN | 10 |
commence; business | START | 2 |
commence; meeting | BEGIN | 17 |
commence; meeting | START | 9 |
commence; day | BEGIN | 8 |
commence; day | START | 3 |
This time we will not expand Table 6 - it contains a total of 81 learning events! Rather, we will present only the first ten learning events, as they would appear after such table expansion and randomization (like we did in the previous exercises).
Table 7
Trial | Cues | Outcomes |
1 | commence; meeting | BEGIN |
2 | commence; movie | START |
3 | commence; meeting | BEGIN |
4 | commence; day | BEGIN |
5 | commence; meeting | BEGIN |
6 | commence; machine | START |
7 | commence; movie | START |
8 | commence; machine | START |
9 | commence; machine | START |
10 | commence; movie | START |
... | ... |
Finally, Table 8 represents the value of the learning weights as they get updated for each cue-outcome combination, for the first ten and the last three learning events.
Table 8
Trial | commence | business | day | journey | machine | meeting | movie | |||||||
Outcome | Outcome | Outcome | Outcome | Outcome | Outcome | Outcome | ||||||||
BEGIN | START | BEGIN | START | BEGIN | START | BEGIN | START | BEGIN | START | BEGIN | START | BEGIN | START | |
1 | 0.010 | 0.000 | 0.000 | 0.000 | 0.000 | 0.000 | 0.000 | 0.000 | 0.000 | 0.000 | 0.010 | 0.000 | 0.000 | 0.000 |
2 | 0.010 | 0.010 | 0.000 | 0.000 | 0.000 | 0.000 | 0.000 | 0.000 | 0.000 | 0.000 | 0.010 | 0.000 | 0.000 | 0.010 |
3 | 0.020 | 0.010 | 0.000 | 0.000 | 0.000 | 0.000 | 0.000 | 0.000 | 0.000 | 0.000 | 0.020 | 0.000 | 0.000 | 0.010 |
4 | 0.030 | 0.010 | 0.000 | 0.000 | 0.010 | 0.000 | 0.000 | 0.000 | 0.000 | 0.000 | 0.020 | 0.000 | 0.000 | 0.010 |
5 | 0.039 | 0.010 | 0.000 | 0.000 | 0.010 | 0.000 | 0.000 | 0.000 | 0.000 | 0.000 | 0.029 | 0.000 | 0.000 | 0.010 |
6 | 0.039 | 0.020 | 0.000 | 0.000 | 0.010 | 0.000 | 0.000 | 0.000 | 0.000 | 0.010 | 0.029 | 0.000 | 0.000 | 0.010 |
7 | 0.038 | 0.029 | 0.000 | 0.000 | 0.010 | 0.000 | 0.000 | 0.000 | 0.000 | 0.010 | 0.029 | 0.000 | 0.000 | 0.020 |
8 | 0.038 | 0.039 | 0.000 | 0.000 | 0.010 | 0.000 | 0.000 | 0.000 | -0.001 | 0.020 | 0.029 | 0.000 | 0.000 | 0.020 |
9 | 0.037 | 0.048 | 0.000 | 0.000 | 0.010 | 0.000 | 0.000 | 0.000 | -0.001 | 0.029 | 0.029 | 0.000 | 0.000 | 0.020 |
10 | 0.037 | 0.058 | 0.000 | 0.000 | 0.010 | 0.000 | 0.000 | 0.000 | -0.001 | 0.029 | 0.029 | 0.000 | -0.001 | 0.029 |
... | ... | ... | ... | ... | ... | ... | ... | ... | ... | ... | ... | ... | ... | |
79 | 0.208 | 0.295 | -0.003 | 0.016 | 0.060 | 0.011 | -0.003 | 0.016 | -0.012 | 0.091 | 0.121 | 0.035 | 0.045 | 0.126 |
80 | 0.205 | 0.302 | -0.003 | 0.016 | 0.060 | 0.011 | -0.003 | 0.016 | -0.012 | 0.091 | 0.118 | 0.042 | 0.045 | 0.126 |
81 | 0.212 | 0.297 | -0.003 | 0.016 | 0.060 | 0.011 | -0.003 | 0.016 | -0.012 | 0.091 | 0.118 | 0.042 | 0.052 | 0.122 |
The whole learning session can be presented visually:
The figure reveals some interesting results of learning.
First, it appears that the context of <commence> gives a bit more support to the outcome START than to BEGIN. This follows from the fact that <commence> was present on each trial, and, overall, START was somewhat more frequent than BEGIN (it was used in 46 vs. 35 trials). We can also see that the association weight between <commence> and BEGIN shows a tendency to stabilise (that is: reach asymptote) around the value of \(0.2\). This is not the case for the weight between <commence> and START, which continues to grow in strength till the end of the learning session.
Next, our machine learned that <machine> and <movie> have a tendency to START, but that a <meeting> most likely BEGINs. There are some other, less strong positive connections, which reveal the 'doubts' that our machine has; for example, sometimes a <movie> can BEGIN and a <meeting> can occasionally START but a <machine> will most definitely not BEGIN, since that association weight terminates with a small negative value.
Linguists will recognize in this description of learning the dimensions that are typically distinguished in corpus-linguistic approaches to lexicography. This parallelism illustrates nicely how a computational modelling approach can be directly relevant to core questions in linguistics while adding insights as to how learning the relevant dimensions of lexical experience are identified and learned over time. Crucially, this approach works on much more than lexical phenomena and we refer to our Outputs page for other examples.
Petar/Dagmar